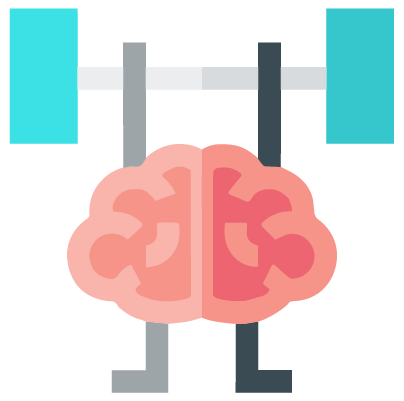
Tangible AI
An informed discussion about AI requires an understanding of the technologies used.
Artificial intelligence (AI) is a topic that currently raises many hopes, but also fears. The abstract methods an AI uses to make decisions can be very different from normal human thinking and are difficult for many to grasp. In fact, many AI models represent a “black box” even for their developers, and the decisions, although apparently correct, are difficult to understand and verify.
Inclusive teaching approaches ensure the participation, understanding and benefits of AI for everyone.
The aim is to create an intuitive understanding of AI through experience and to make its possibilities and limitations tangible through interactive AI prototypes and demonstrators. Meaningful visualizations help to gain a look “into the AI models”. At the same time, awareness-raising, analysis and solution processes should also be used to support the developers of AI solutions in integrating experience and explainability into their models and thus making them more transparent, robust and fair.
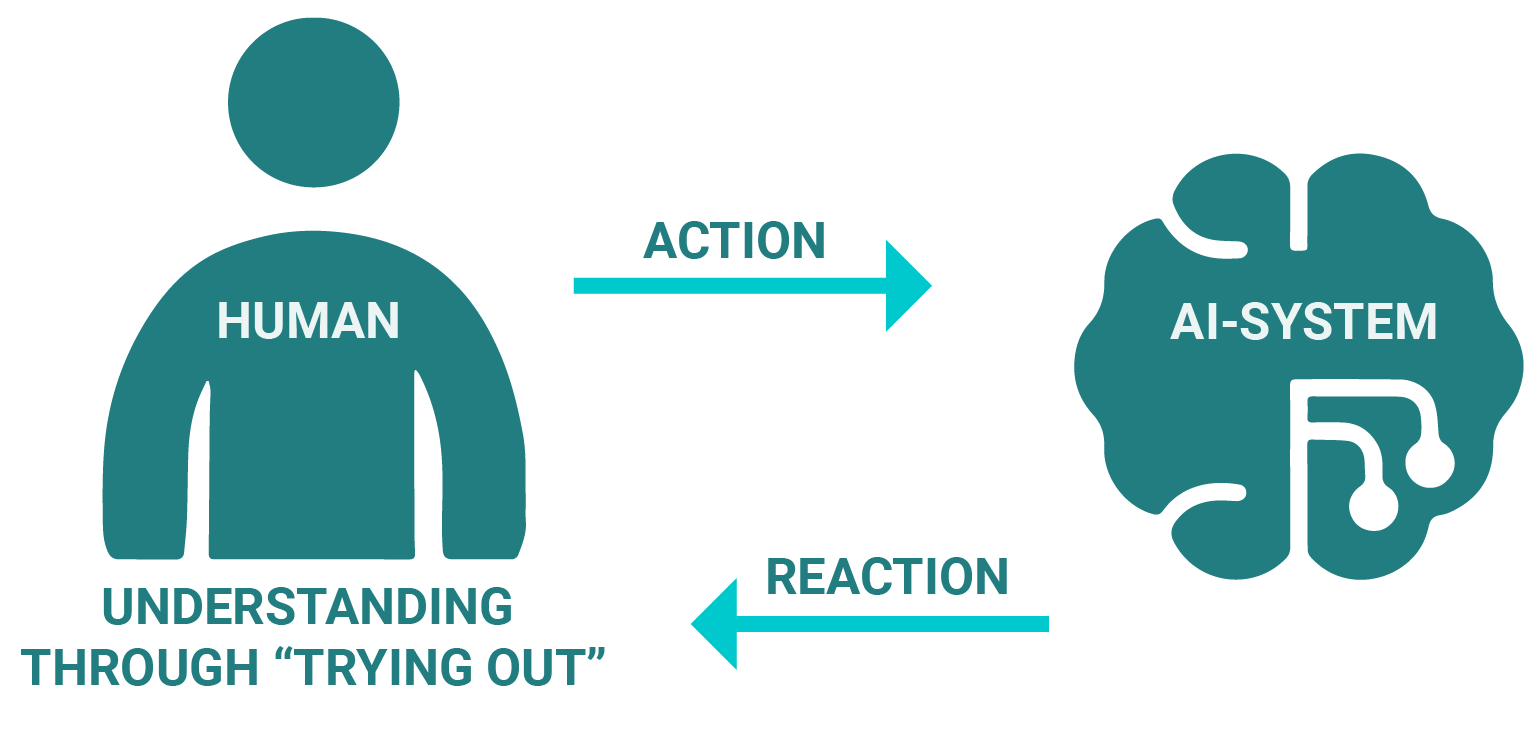
Interaction
Various prototypes for “hands-on AI” are to be developed.
These are made available both on the premises of the ZEKI (Center for Tangible AI and Digitalization) and online. They convey the possibilities, but also the risks, of using AI, and thus serve to reduce fears of contact and provide the basis for an informed, conscious use of AI in everyday life.
The demonstrators are intended to encourage both AI users and developers to question AI decisions:
Why was this decision made and why not another?
When is the decision right and when is it wrong?
Can I trust the decision?
Is the decision fair?
Explainability
The goal of explainability is the controlled and regulable design of AI.
To this end, the project is working on various methods and solutions for Explainable Artificial Intelligence (XAI) that can be used to better understand AI decisions and can be applied to a wide range of AI methods. The knowledge gained will be shown in workshops and courses, among other things, and prepared in appropriate accompanying materials.
Results
The topic of XAI can be overwhelming for people who do not have a deep understanding of AI. Our XAI tutorial provides a simple and comprehensive introduction to XAI. The possibilities, but also the limitations of XAI are presented in a practical way.
Finding an XAI method and creating an explanation for an AI system can be difficult. The XAI MethodFinder helps to find an XAI method for specific use cases. Based on the target group, technical details of the AI system and preferences for the explanation of suitable XAI methods are recommended to the user. In addition, the user also receives assistance and tips on how the method should be applied in a way that is as understandable as possible for the target group.
If you would like to learn more about the various XAI methods and find a suitable XAI method for your AI system, we recommend our XAI factsheets. XAI methods are presented there in simple language and their possible applications are listed.
Label-free Activation Maps (LaFAM) is a method developed as part of the Go AI project for models that are trained in self-supervised environments. This means that such models have no labels, so that traditional methods are not applicable.
Resources
- XAI Method Cards (German) PDF
- XAI Method Cards (English) PDF
- XAI Demonstrations: https://gt-arc.github.io/go-ki-demo/